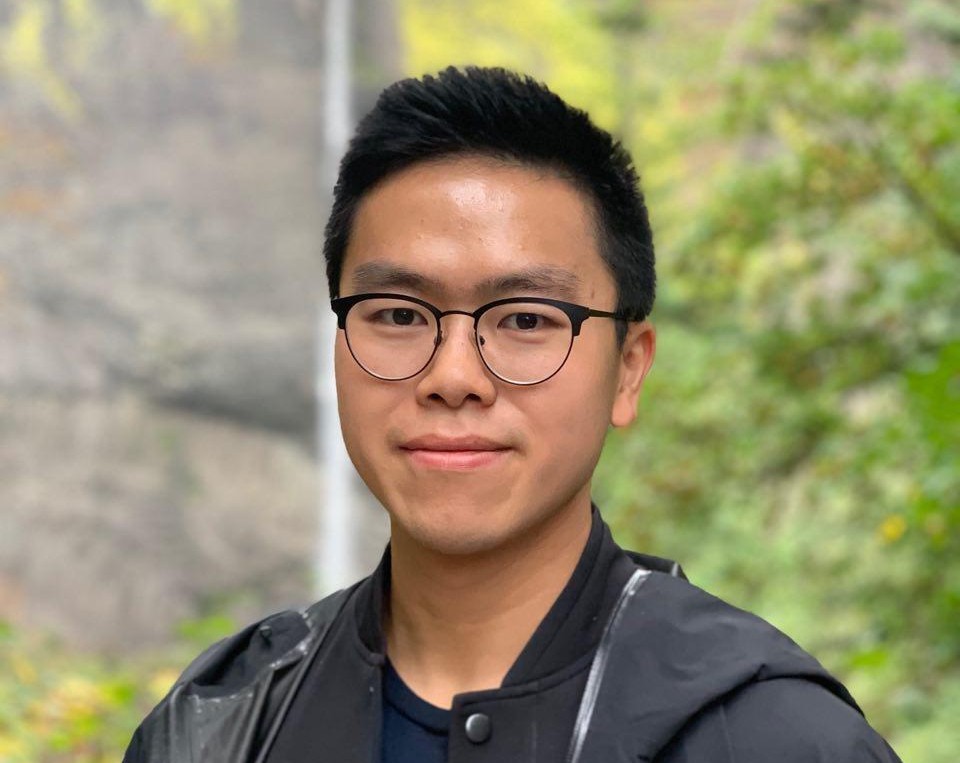
At the same time, privacy regulations like GDPR and CCPA have added layers of operational complexity, demanding stricter governance and compliance measures.
By Alfred Sin, Head of Personalization, Amperity
The technology is ready. The customer expectations are clear. The only question left: Will your brand lead the transformation or end up playing catch-up?
The Evolution of Customer Data Management
AI-powered CDCs are already driving results in the real world:
Imagine a world where your brand anticipates customer needs before they’re even expressed. A customer browses winter coats on your mobile app, and by the time they visit your website, they’re greeted with personalized recommendations for matching accessories with pricing adjusted to their typical spending patterns. This isn’t science fiction; it’s the reality that AI-powered Customer Data Clouds (CDCs) are making possible today.
Keeping Pace with Evolving Regulations: Privacy laws are rapidly changing. A proactive stance is key: invest in privacy-enhancing technologies, establish strong data governance, and maintain transparency with customers about how their data is used.
The rapid advancement of AI is reshaping how brands interact with customers. In our digital-first world, traditional approaches to customer data management simply can’t keep pace. Brands can no longer rely on fragmented systems and basic segmentation tactics, they need intelligent, unified platforms that maximize AI’s capabilities for deeper insights and real-time decision-making.
Four AI Trends Reshaping Customer Data Strategies
Despite the upside, implementing AI-driven CDCs comes with challenges that require thoughtful strategy:
- Democratized Data Access: AI is lowering the barrier to data analytics. Now, non-technical employees can query data, generate insights, and answer critical questions using natural language. This opens the door for wider participation in data-driven decision-making across the organization.
- Predictive AI for Proactive Engagement: The real power lies in anticipation. AI-powered CDCs predict churn risks before customers leave, suggest what they’re likely to purchase next, and deliver personalized recommendations at precisely the right moment, enabling brands to shift from reactive to proactive engagement.
- Privacy-First AI Models: With growing consumer concern and tightening regulation, privacy-enhancing technologies are essential. Leading CDCs now integrate privacy-forward AI techniques, enabling brands to personalize responsibly while meeting compliance standards.
- AI Agent-Powered Omnichannel Personalization: Customer journeys are no longer linear. Modern consumers expect fluid, personalized experiences across apps, websites, emails, and service channels. AI agents within CDCs automate these journeys, ensuring consistency and relevance at every touchpoint.
Navigating the Challenges of AI-Powered Data Strategies
AI-powered CDCs aren’t a futuristic ideal, they’re the present reality for leaders in customer experience. Brands that move now will define the standard for personalization, trust, and data-driven growth.
Balancing Personalization and Privacy: Hyper-personalization can create value, but if misused, may come off as invasive. Brands must respect preferences, offering opt-ins and contextual personalization that feels helpful, not overbearing.
Brands that address these head-on won’t just mitigate risk, they’ll unlock competitive advantage.
AI-powered CDCs have changed the game. These platforms not only unify data sources, but also enable insights to be turned into action instantly. Unlike legacy CDPs, which often require custom integrations and force trade-offs between batch and real-time data access, CDCs operate natively in the cloud, delivering immediate accessibility when and where it’s needed.
Real-World Transformation Across Industries
Unlike traditional Customer Data Platforms (CDPs) that focus primarily on consolidating data, CDCs are designed for action, delivering real-time insights, AI-powered automation, and seamless integration across every customer interaction. Their cloud-native architecture breaks down data silos, transforming raw information into actionable insights to drive personalized customer experiences at scale.
- Retail & E-Commerce: A global retailer unified customer profiles, merged duplicate loyalty accounts, and launched AI-driven reactivation campaigns while increasing retention by 18%.
- Hospitality & Travel: An international hotel group unified reservation, loyalty, and sentiment data to personalize upgrade offers, driving a 24% increase in ancillary revenue.
- Automotive: Automakers are using CDCs to anticipate maintenance needs, personalize service suggestions, and tailor lease-end offers based on individual driving behavior while improving long-term loyalty.
Your Strategic Roadmap to AI-Powered Personalization
The opportunity to lead with AI-powered CDCs is now. To make the most of it, brands should:
- Align Tech Investments to Business Goals: Define your top personalization use cases before investing in platforms. When tech directly supports business goals like CLV growth or churn reduction, you’ll see faster ROI.
- Build AI Fluency Across the Org: The best platform won’t succeed without people who know how to use it. Build cross-functional AI literacy so that teams at every level can translate insights into action.
- Keep the Customer at the Center: Use AI to augment and not replace human judgment. Personalization should always aim to enhance customer value and earn trust.
- Start Small and Prove Value: Don’t wait for a full-scale rollout. Run small pilots, track outcomes, and iterate. Quick wins can build momentum and stakeholder confidence.
The Future Is Already Here
For years, brands have struggled with fragmented customer data, leading to incomplete insights and reactive engagement strategies. Instead of proactively guiding customers through meaningful journeys, many companies remain stuck asking retrospective questions, like why a customer lapsed.
As AI becomes central to CDCs, four key trends are transforming how businesses engage with data:
To manage this, early efforts relied on basic rule-based segmentation, which grouped customers by broad characteristics. While sufficient in the past, this method falls short today while unable to account for real-time behaviors or rapidly shifting preferences.