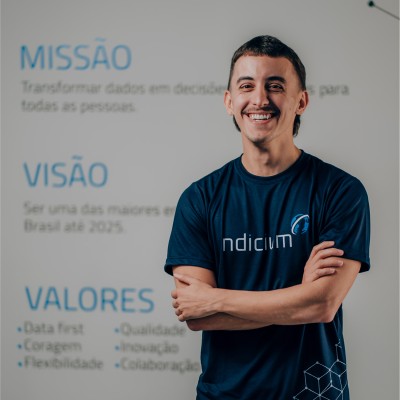
It has been about two years since generative AI technology exploded onto the radars of most businesses following the release of ChatGPT in late 2022. In that time, the vast majority of organizations have embraced genAI technology at least to some extent. Gartner found that as of January 2024, nearly two-thirds of businesses were using genAI, a number that has almost certainly only increased since that time.
Basic use cases are ones where businesses adopt off-the-shelf genAI technology to streamline routine tasks. For example, they might use Microsoft 365 Copilot to help draft emails, or use generative AI coding tools to write source code for simple applications.
Allow me to begin by making clear what I mean when I talk about basic vs. advanced generative AI use cases.
To that end, keep reading for guidance on how to take your business’s generative AI strategy beyond the basics and integrate the technology into all aspects of your operations. These tips reflect my experience working with businesses across the globe over the past couple of years to build the data foundations necessary to take full advantage of genAI, then implement genAI solutions that extend well beyond simple use cases.
Basic vs. advanced genAI use cases
Yet, just because the typical business is now using generative AI in some way hardly means that it’s living fully in the genAI era. Generative AI use cases vary widely, and many organizations remain limited to genAI solutions that merely scratch the surface of the technology’s full potential.
By David Eller
In addition to having the right engineering talent at your disposal, advanced genAI use cases also require adopting a product management mindset. In most cases, businesses won’t know exactly how their employees will use next-generation genAI tools, or precisely what their needs will be, until the solutions have gone through multiple iterations of development and experimentation.
The good news is that organizations don’t have to build a genAI “dream team” in house. Outsourcing genAI data engineering and model development is becoming a common practice. While large enterprises may opt to hire their own genAI talent, smaller organizations can pursue advanced genAI use cases effectively with help from external experts.
- Personalized customer support: With genAI, customer support teams can automatically craft personalized responses to clients, allowing them to respond more quickly without sacrificing quality or personal touch.
- Data analysis: Using genAI models trained on custom business data, employees can ask questions about data in natural language, then have the models respond with natural-language answers that employees can readily understand. This “democratizes” data analytics and makes it possible for anyone in the business, not just data analysts, to make data-driven decisions.
- Contact generation: Businesses that need to produce custom contracts for customers can have genAI draft the documents, saving substantial time and freeing employees to focus on other, more productive tasks.
- Legacy code translation: GenAI tools that are customized to understand specialized code bases can automatically modernize legacy application code, allowing software development teams to refactor applications with much less time and effort.
There’s no denying that implementing the next generation of genAI tools and services will require a lot more skill, effort and product development than more basic genAI. But this is the challenge that the typical organization today needs to tackle to retain a competitive edge through genAI.
How to implement advanced genAI technology
In the long run, organizations that are able to gain the greatest competitive edge from generative AI will be those that go deeper – that use genAI to do much more than generate boilerplate code or write emails, for example.
This means that rather than hiring engineers to build advanced genAI solutions and then foisting them upon users, businesses should expect to undergo a process where developers and target users can interact to validate features and ensure that the end product actually matches user needs.
A year or two ago, the key question for businesses to ask themselves was whether they were using generative AI in some way. Today, the ground has shifted, and the question has become whether organizations are expanding beyond simplistic genAI use cases by pursuing more advanced solutions.
These are examples of generative AI use cases that move the needle much further – and they’re exactly the types of opportunities businesses will need to pursue if they want to realize the full value of genAI. Organizations that use generative AI only to target low-hanging fruit won’t gain the efficiencies they need to stay competitive against businesses that take genAI to the next level.
The challenge with taking genAI to the next level, of course, is that it requires more than adopting off-the-shelf genAI tools and services. To enable advanced use cases, businesses need custom models, as well as a data platform that allows them to connect models to the information they need to understand the organization’s unique requirements.
Enabling the next generation of genAI
And to build and deploy those models and the data that powers them, you need a team of experts who understand the complexities of genAI technology and the data science behind it. Otherwise, you’re likely to end up with projects that are among the 30 percent of genAI initiatives that, according to Gartner, will fail due to issues like poor data quality and risk management.
However, there are a whole host of other genAI use cases that allow businesses to leverage the technology in much more powerful ways. For example, here’s a small sampling of ways in which organizations can infuse genAI into core business processes:
There’s certainly nothing wrong with using generative AI for tasks like these. This breed of genAI solutions is easy to implement, and it brings real value to the organization.