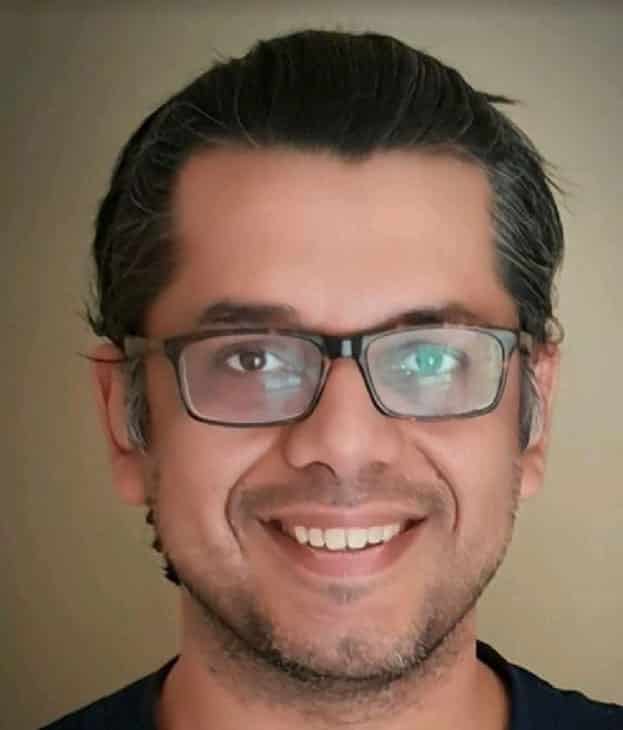
Training data bias could also lead to a variety of security dangers, and for one good reason. Most of the AI databases, even the ones related to cybersecurity, can experience what we call “cultural biases.” They can easily detect malware coming from an English-speaking country and counter it for most of its course. However, when the threat came from a non-English-speaking country, there were many blind spots that the threat detection algorithm could not cover. This allowed the malware to seep into the system unnoticed, and it continued until the training data was culturally diversified.
Interview:
Impact of Training Data Bias: Given that training data bias can cause AI systems to misinterpret or overlook cyber threats, could you provide an example where this form of bias led to a security breach or significant oversight in threat detection?
From the very start, bias happens because there is a gap in the developing team’s knowledge, causing the AI database to be incomplete and potentially inaccurate. Even the most skilled team won’t be able to know every aspect of the world, which means there will undoubtedly be gaps at some point. By collaborating with academia, industries, and cybersecurity communities, we can fill those database gaps and potentially reduce AI bias. For instance, collaboration with an academic institution gained us access to technology transfers, joint research projects, and even talent exchanges. This helped us create robust, bias-free cybersecurity solutions that were much more effective.
Algorithmic Bias Challenges: How do algorithmic biases in AI models specifically impact the effectiveness of cybersecurity measures, and what steps are taken to mitigate false positives and false negatives that result from these biases?
Future of AI in Cybersecurity Amidst Bias Concerns: Considering the challenges of AI bias, how do you envision the future role of AI in cybersecurity? Will there be a shift towards more human-centered approaches, or will AI continue to dominate with improved bias mitigation techniques?
AI bias can pose a specific threat to cloud security, mainly because it’s a bit more challenging to look for it here. It can miss or inaccurately detect threats due to its incomplete training data, lead to alert fatigues that overwhelm our security team, and leave it vulnerable to emerging threats. By educating the team about diversity and accounting for cloud-specific peculiarities, it would become much easier to detect threats. For example, we would implement bias mitigation techniques such as robust testing, using new and historical data to identify a potential bias. This would make the cloud platform more resilient, enhancing user data protection.
Advice for Emerging Cybersecurity Professionals: Finally, for emerging cybersecurity professionals concerned about AI bias, what advice would you offer to help them navigate and contribute positively to this evolving challenge?
Ethical Considerations and AI Bias: How does your company address the ethical considerations that arise from AI bias, especially when it comes to ensuring that AI-driven cybersecurity tools do not inadvertently perpetuate societal biases?
By Randy Ferguson
If you are concerned about AI bias, the best thing you can probably do is stay informed. Developments are made daily, and with them, there is a new opportunity for bias. You need to stay one step ahead and train your algorithm to see these new changes, thoroughly testing for them. Collaboration is important as a low-bias AI algorithm is diverse in its data pool. You should encourage AI collaboration instead of human replacement to make it through this shift with as few breaches as possible. AI algorithms are good, but their lack of intuition and adaptive abilities can lead to bias and security issues.
Mitigating AI Bias: What strategies or methodologies are currently in place to identify and mitigate AI biases within your cybersecurity operations? Are there specific tools or practices you find most effective?
AI Bias and Cloud Security: With cloud security being a major concern, how has AI bias specifically posed threats to cloud environments, and what examples can you provide where addressing AI bias improved cloud security measures?
Khurram Mir, the Chief Marketing Officer at Kualitatem, brings a profound depth of knowledge and experience to the forefront of cybersecurity and software quality assurance. With a solid educational foundation, having earned a bachelor’s degree in computer sciences followed by an MBA, Khurram has crafted a career that seamlessly bridges the technical and strategic domains of cybersecurity. At Kualitatem, a company dedicated to independent testing of software development processes, he leads a team committed to enhancing data security and software integrity through meticulous testing and audits. His expertise encompasses a wide array of critical functions, from requirements validation and test case writing to detailed reporting and test plan development. Khurram’s approach is deeply rooted in addressing the core challenges faced by customers, particularly in an era where digital threats pervade every aspect of technological development. His insights are particularly relevant today, as he discusses the integration of AI into cybersecurity, highlighting how advanced technologies can be harnessed to fortify digital defenses while also navigating the complex landscape of software testing and data protection. This conversation aims to unpack these intricate themes, offering clarity and forward-thinking strategies to our audience.
Algorithm biases can significantly compromise the effectiveness of cybersecurity, as it relies heavily on data that has been used for its training. If there are flaws in that data or it is incomplete, it could lead to inaccuracies in the threat detection and false positives or negatives. To prevent this from happening, it’s important to curate and validate the data regularly. Human intelligence should be used in this case, as it can identify potential biases that could otherwise compromise an algorithm.
Collaboration and AI Bias Research: How important is collaboration between academia, industry, and cybersecurity communities in researching and addressing AI bias? Can you highlight any successful partnerships or projects that have made significant strides in this area?
Cognitive Bias in AI Tuning: Can you discuss a scenario where cognitive bias influenced the outcome of AI-driven cybersecurity efforts, particularly in how models were created, trained, or fine-tuned?
To prevent our cybersecurity tools from inadvertently perpetuating societal biases, we often take a holistic approach to cover all blind spots. Education and training of the human counterparts is essential here, encompassing everything from the development to the deployment of these tools. This is further used to train the AI algorithm with vast amounts of data, implementing techniques like fairness testing to monitor for biases. We also implement a variety of ethical standards and guidelines based on transparency and accountability in the development process.
The main problem with AI is that it can only see what it has been trained to. It has a database of threats to which it knows how to respond, which means it might not know how to react to the newer threats. Hackers are becoming more ingenious every day, modifying their attacks to be similar but different somehow. The AI system will recognize and react to some of those attacks, but it will only be partial. Without human involvement, increased reliance on AI could lead to target misidentification and false positives or negatives.
When the AI training team is biased, there is a good chance that the AI database shares the same “quality.” Let’s say you are developing an AI malware program to prevent criminals from accessing your database. You have the computer skills to put the algorithm in motion, but you lack the specific knowledge of criminal behavior and cyberattacks. This could lead to an incomplete data pool that can overlook or misinterpret an upcoming attack. It may detect the standard attacks, but if it was made using specific evasion techniques, it could lead to a security breach.
We use different methods and strategies to find and mitigate AI bias, the most important being thorough testing and validation. We cross-reference the datasets with the emerging trends, conducting regular tests to reduce false positives or negatives. Aside from constantly updating and diversifying the data, we also maintain human oversight to improve its performance. No matter whether the AI system detects a threat or not, our human analysts are in a continuous feedback loop to reduce the limitations of these biases.
It’s difficult to say if there will be a shift towards a human-centric approach, as AI involvement significantly improved the process by offering what we call a foundation. That said, the existence of this bias makes it improbable that companies would be able to fully rely on this technology. Instead, it’s more probable that AI will be used to create the structure of the algorithm, with human intelligence continuously monitoring and testing for biases. A future where only one is used is unlikely, but one where both are balanced is quite probable.
Introduction to AI Bias in Cybersecurity: Can you elaborate on how AI bias has become a significant threat in the cybersecurity domain, especially with the increased reliance on AI for threat detection and response?