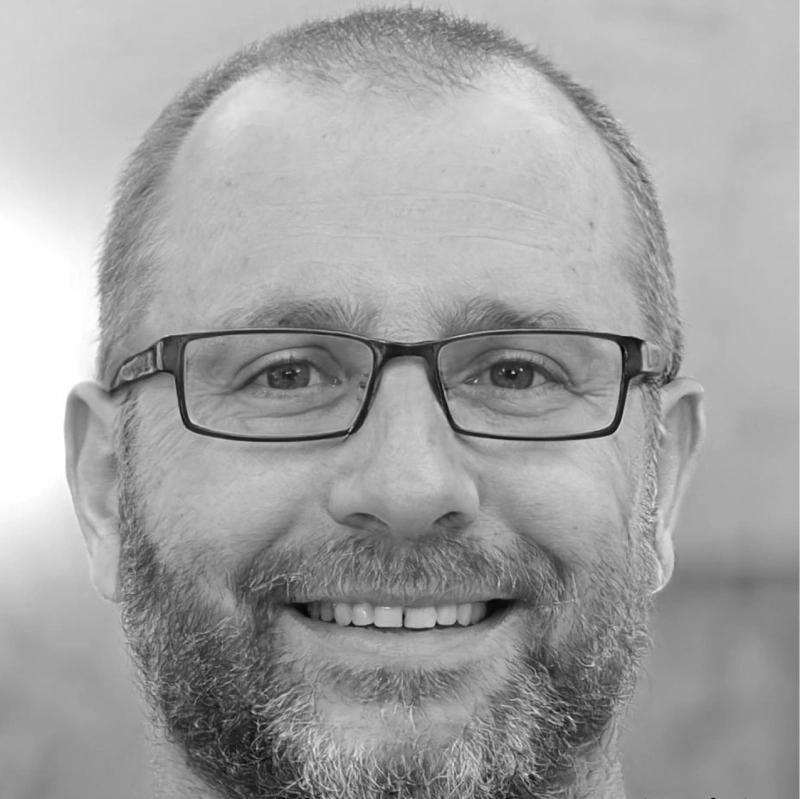
When integrating AI into existing workflows, collaboration across departments is key. How do you suggest aligning IT, legal, and marketing teams that often work at different speeds and with different priorities?
Speed is often prioritized over security in fast-moving industries. What’s a better framework or mindset companies can adopt to ensure that AI adoption doesn’t outpace necessary cybersecurity and legal reviews?
Read on as Karthi shares his thoughts on the current AI landscape, its impact on business operations, and how organizations can navigate its complexities.
Dynamic Creative Optimization (DCO) is rapidly becoming a favorite in omnichannel strategies. How do you recommend companies balance DCO with the creative instincts of human marketers?
An AI-powered chatbot can generate unfitting or off-brand messages without human intervention. An AI tool can start generating offensive messages with a biased dataset used for training. An AI-driven advertisement campaign may lead to PR backlash due to misinterpreting sarcasm on social media. All these issues can escalate quickly before detection without human oversight.
The marketing teams and other business teams may see IT as “slowing down” the speed to market. The legal team adds more complexity to the mix when project timelines are not properly planned and expectations are not clearly set. It’s beneficial for organizations to create “cross-functional AI working groups” that meet regularly to achieve the AI project goals.
You mention that AI should not be left “to its own devices.” Can you share an example (real or hypothetical) of what can go wrong when human oversight is minimized in an AI-powered marketing campaign?
By Randy Ferguson
In order for mid-sized businesses to ensure diversity across gender, ethnicity, geography, and socioeconomic factors, they should begin by auditing their training datasets for validity. Next, during model training, they should apply bias-detection checkpoints and construct an internal AI ethics committee to overlook active use cases.
To retain human oversight, companies should use DCO to test variations differently while setting brand voice, emotional resonance, and creative themes. To put it simply, DCO is an enhancer for verified creative thinking, not a stand-in for intuitive storytelling.
For companies just starting their AI journey, starting small seems to be a recurring theme. What are a few “low-risk, high-reward” AI applications that businesses can test to build confidence and gain early wins?
The low-hanging fruit is AI-powered chatbots to service customers by addressing the frequently asked questions that do not need a lot of human intervention. Another area to start quickly is automated testing for marketing campaigns. AI features can be applied to the existing “Customer Data Platforms” of the organization, which will be another quick win.
For companies to evaluate their data quality, governance structures, technical skill sets, and management maturity, they need to conduct a “readiness assessment.” One efficient method is for companies to pilot small AI initiatives and observe where bottlenecks develop, whether it’s in model interpretation, data access, or organizational buy-in to help uncover areas of capability deficiencies organically.
You’ve highlighted that effective AI use demands more than just plugging in new tools. How can companies realistically assess their internal capability gaps before adopting AI, especially when they don’t yet know what they don’t know?
A dynamic contributor to community initiatives and knowledge-sharing forums, Karthi is a strong advocate for fostering collaboration and continuous learning in the tech space. In this interview, we dive into Karthi’s insights on the “The Tempting Benefits and Hidden Pitfalls of Integrating AI Technology,” his recent article that sheds light on the rapidly evolving trends of AI integration. Karthi explores the promises AI holds for transforming businesses and the potential risks that companies should be mindful of during implementation.
Personalization addresses individuals according to demographics and behavior. Humanization takes it a step further–it’s all about making the content feel as if it’s genuinely empathetic, relatable, and emotionally intelligent. The variation matters because AI has the risk of producing ‘personalized spam’ without humanization that feels forced and emotionless instead of building sincere relationships with customers.
Much has been made about the importance of personalization in AI-driven marketing. In your view, what’s the difference between personalization and humanization of AI content, and why does that distinction matter?
The common misconception is that AI is a “plug and play” solution. Leaders should understand that AI is not here to replace human roles but to complement them in enhancing processes. In reality, “AI is a journey, not a tool”.
Bias in AI is a growing concern. What practical first steps can a mid-sized business take to ensure their AI training data and algorithms are both ethical and inclusive from the beginning?
Time savings and precision are almost always celebrated, and though these may be efficient advantages, the most overlooked one is AI’s ability to unlock hidden customer insights at scale. When AI is properly implemented, it doesn’t just automate. It identifies behaviors and patterns that human analysis might otherwise miss. It can reveal unmet customer needs and blossoming market trends before competitors have a chance to even spot them.
In this email interview, we have connected with Karthi Gopalaswamy, who brings over 20 years of expertise in enterprise architecture, SaaS solutions, and digital transformation.
Let’s start with the big picture. AI is often praised for its ability to reduce time and increase precision, but in your experience, what is the most overlooked advantage of implementing AI in business operations – particularly marketing?
It’s critical for companies to adopt a “secure by design” approach, which combines security, legal, and compliance reviews in the AI project lifecycle. The best mindset is, “If it’s not safe, it’s not fast”. The short-term gains can erode quickly when security vulnerabilities pop up later. A phased rollout, testing the AI in controlled environments before full deployment is the right methodology.
Finally, looking ahead…What do you think is the biggest misconception business leaders have about AI today, and what mindset shift do you believe is necessary for long-term success?